- Like Resource
The three dimensions of successful marketing mix modelling and ROI measurement
-
Client:
-
Industry:
Key highlights
How The Smart Cube helps CPG businesses optimise marketing strategies in the face of unpredictable demand
Warning: Trying to access array offset on value of type bool in /sites/smartcube.idstaging.co.uk/files/web/app/themes/smartcube/functions.php on line 1017
Warning: Trying to access array offset on value of type null in /sites/smartcube.idstaging.co.uk/files/web/app/themes/smartcube/functions.php on line 1017
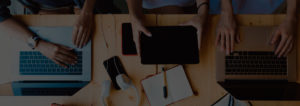
Introduction
Marketing Mix Modelling (MMM) is by no means a new subject, but in increasingly uncertain and fast-changing business environments, the parameters around it are moving at breakneck speed. Today, having the ability to understand growth drivers and develop informed strategies based on scientific evidence is a must. It’s the only way for commercial teams and advertisers to adjust trade and media strategies and respond to the changing circumstances we continually face.
A good MMM strategy can help organisations to determine the effectiveness of marketing campaigns, better allocate budgets, and provide decision-makers with the ability to test potential scenarios and strategies with accuracy. In turn, this can result in improved brand loyalty, increased trust, and better ROI
However, with rapidly changing consumer behaviours, organisations are faced with the challenge of responding quickly and adapting their trade and marketing strategies to emerging trends. All while ensuring processes are regular, repeatable, and scalable in order to meet both short and long-term growth objectives
In this white paper, we discuss the three main dimensions that combine to form a complete MMM solution – and share the best practices for designing a marketing mix strategy for the modern age that optimises ROI. We’ll also discuss how The Smart Cube’s mix of artificial and human intelligence, comprising in-house and external support, can help you optimise your practices
An introduction to marketing mix modelling
The concept of a marketing mix strategy was first introduced in 1960 by Edmund Jerome McCarthy, described by the Oxford Dictionary of Marketing as a “pivotal figure in the development of marketing thinking.” McCarthy coined the four Ps of marketing; product, price, promotion, and place – the elements widely covered by marketing mix modelling initiatives today.
The key purpose of marketing mix modelling is to understand how the different factors affecting a marketing mix will impact results. It provides actionable intelligence that can be used to assess the effectiveness of all marketing levers, better allocate budgets, more successfully MMM solutions are developed using multivariate econometric modelling techniques with the objective of isolating the impact of marketing activities and promotions from baseline sales.
Adopting such a solution is a big step up from simply tracking KPIs and undertaking customer research surveys. Instead of seeing how many clicks a marketing campaign has received, for instance, MMM allows you to gain true visibility into how those clicks translate into sales.
It’s especially useful for companies that need to perform better with reduced budgets, those that want to evaluate how a change of strategy might play out, and those that have to publicly report media ROI.
The science behind the insightMMM solutions are developed using multivariate econometric modelling techniques with the objective of isolating the impact of marketing activities and promotions from baseline sales.
The relationship between sales and drivers can be linear or nonlinear. Typically, marketing levers such as TV Gross Rating Points (GRP) or digital impressions have a non-linear relationship with sales. In order to identify the most suited functional form, initial exploratory data analysis, including univariate, bivariate, and correlation analysis with different variable transformations, helps define the hypothesis around response curves. The three most popular regression techniques used for market mix modelling are Linear Regression, Semi-Logarithmic or Log-Linear, and Logarithmic or Log-Log Regression. The choice of the functional form depends on how stable the business environment is and what type of relationships exist among the explanatory variables – for instance, whether each driver is independent of one another or if there is interaction and influence between them. Market mix models are multifaceted, often including the interdependencies within marketing mix variables, immediate vs. lagged responses, diminishing returns, the carryover effects of media (Ad stock), and the effects of competitive marketing activities. It is therefore recommended to consider multiplicative forms of the model, such as Log-Linear or Log-Log, to capture these synergies and reveal interaction effects within the ecosystem. Developing a robust MMM model requires an iterative approach along with a healthy combination of science (including AI and machine learning) and human intelligence, because the model is a quantification of category dynamics as they are in the actual market scenario. A close partnership with business teams is often required to understand the nuances of one’s own and one’s competitors’ marketing activities to accurately isolate the individual effects and draw out the required insights and recommendations. |
The modern challenge of marketing mix modelling
Today, commercial teams and advertisers face the constant challenge of adjusting their trade and media strategies to maximise ROI in the face of widespread, unexpected, and hugely impactful market changes. One example of such a change is the shift away from the traditional advertising channels of TV, print and radio, in favour of digital media. In fact, the increasing digitisation of society has changed more than just the way people view content. Today’s digital-first consumers are more expectant and demanding, and more prone to changing brands and switching loyalties, which means pricing and availability can hugely impact purchasing behaviour.
Today’s consumers have an average of 4 paid video streaming services and 2 music streaming services1
Of course, one challenge that has cast a huge shadow over almost all business processes in almost all industries has been the COVID-19 pandemic; a completely unexpected event which has changed everything from demand for products and services to the way people consume media.
As much as COVID seems like a one-off event, nothing could be further than the truth. The pace of change in modern society means there will always be another surprise around the corner, and marketing mix strategies must be capable of coping with those surprises and providing actionable insights in unfamiliar circumstances. Strategies must acknowledge how customers’ day-to-day lives have changed to breed trust and provide reassurance that brands are there to help and support them.
In this environment, the typical yearly or six-monthly insights many organisations rely on aren’t sufficient. Insights need to be available to decision-makers in an ‘always-on’ mode, and they need to be scalable across different regions and markets. This requires incorporating the most advanced techniques, tools, and methodologies – and these, too, are constantly changing.
For this reason, successful MMM activities can be a vast undertaking if handled solely in-house. Along with a constant influx of accurate and actionable data, they require a mix of skill sets, from data engineers and data scientists to front-end developers. This can make developing and scaling models a challenge. Which is why so many organisations choose to build teams from a healthy mix of internal and external resources to accelerate the development of their MMM capabilities in a way that is repeatable and scalable.
Partnering with the right provider in this way can provide some significant advantages. You get to lean on the experience of an organisation that has worked with multiple clients, gaining vital cross-industry, cross-category, and cross-market experience. This, in turn, will bring new ideas and shared perspectives that won’t be present if you work solely with your in-house teams.
In this environment, the typical yearly or six-monthly insights many organisations rely on aren’t sufficient. Insights need to be available to decision-makers in an ‘always-on’ mode, and they need to be scalable across different regions and markets.
Three key dimensions to MMM
Faced with these challenges, a successful marketing mix strategy requires a combination of immediate insights, long-term forecasts, and the ability to react to changing landscapes. The system required to enable this must include skilled people and the right processes, technology, and data. In this section we examine three key approaches and the value they can add to organisations in different situations.
1. MMM models for continuous planning and tracking short-term impacts on a regular basis
Most organisations who have an MMM strategy in place monitor the short-term impacts of their investments, often with six-monthly or even yearly updates. The problem with this is that today, markets and behaviours are changing far more quickly than those updates are made available.
Modern MMM strategies need to be able to measure growth and track ROI in a continuous manner. But generating insights is only half the battle. A truly impactful MMM approach must also contain simulation capabilities, so companies can test scenarios and explore alternate budget allocations to identify opportunities of higher impact. With simulation capabilities, departments can explore possibilities in granular detail, testing which campaigns will perform best, on which channels, at which point in time.
For organisations that require short term insights, the onus must be about making these insights deeper and more regular. This requires a continual cycle of forming strategies, executing them, testing their impact, adapting them, and then repeating that process.
To do this, you need a regular stream of actionable data, the latest analytics capabilities – including simulation and what-if modelling – and a way of presenting those findings to decision-makers in an easily digestible format.
For this reason, an MMM solution can’t be approached as a minor investment or a single role in the organisation. It must either be a dedicated team with enough resources, or the task should be outsourced to a company that has the requisite experience, skillset, data, and technologies on demand.
Modelling must be a continual process of forming strategies, executing them, testing their value, adapting them, and then repeating that process
Use case
The Smart Cube helps a global food and drink leader gain ongoing insight into its marketing mix for snacks
Client
A global food and beverage company with more than 20 different brands and multibillion dollar revenue Challenge
Our client wanted to gain a comprehensive and consistent view of how its marketing strategies were affecting the sales of its savoury snack products. With several product types and brands, the client wanted continuous insight into how different channels, ad types, ad lengths and other factors would impact sales volumes for different products in different regions.
Results
The Smart Cube uses a combination of custom digital technologies and human intelligence (AI+HI) to help our client gain the insights it needs. On an annual basis, we collate and unify a variety of internal and external data on behalf of our client, before using that data alongside linear regression and multiplicative models to isolate the impacts of different volume drivers. This enables our client to:
- Gain continuous insight into the effectiveness of advertising spend and identify key sources of growth
- Optimise marketing mix strategies by product and region to drive higher ROI from campaigns
- Use repeatable, scalable techniques to identify best practices and correct strategies around pricing and distribution
Key facts: How we support a global F&B leader to measure short term ROI impacts
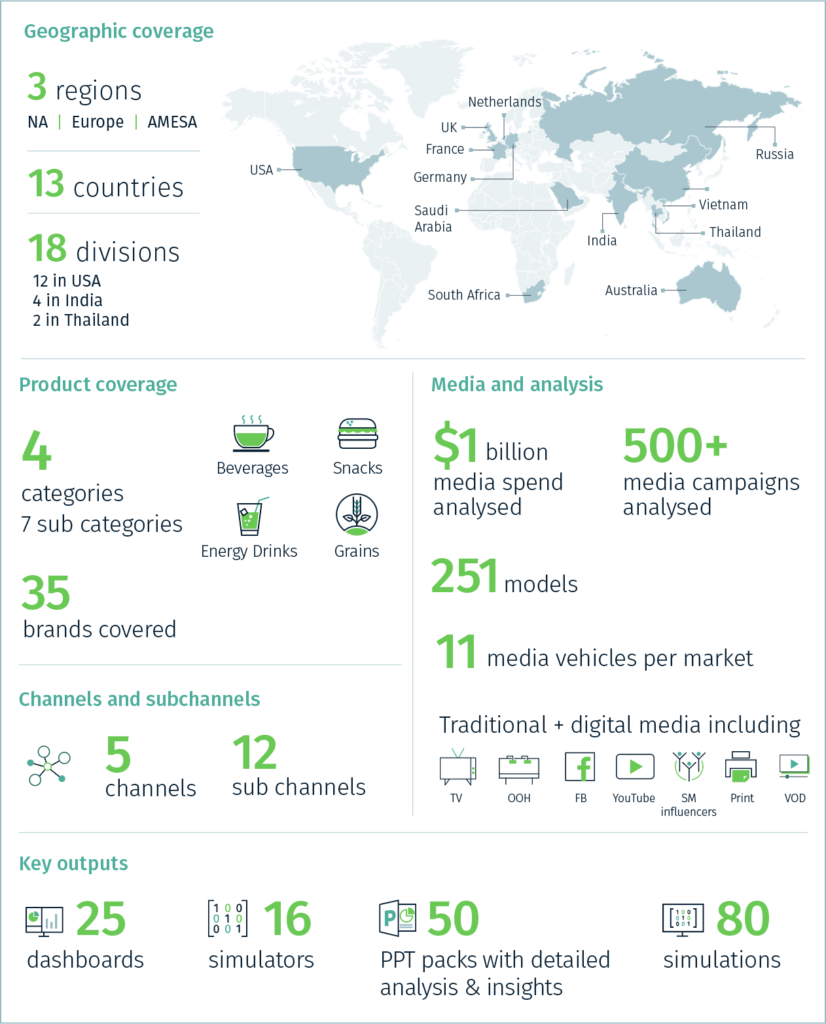
2. Modelling and measuring the long-term impacts of media spend
As important as the short-term impacts of marketing strategies are, it’s not uncommon for companies to rely on them too much. It’s easy to do when metrics are simpler to measure that way, but the true value of a marketing campaign has to be measured over a longer period of time.2
Why are long-term impacts so important? Because marketing strategies should never be solely about quick wins. Instead, they should revolve around building a brand identity and attracting a loyal customer base, which is why the value of building long-term brand equity can’t be overstated. However, as you may imagine, long-term brand equity is a lot trickier to measure than short-term value.
There are lots of ways to examine long-term impacts, though, primarily through modelling or analysing brand metrics, price sensitivity, or activation data. Part of the problem is that measuring long-term impacts requires a data set that spans a long period of time, but there are existing data sets and studies that can guide you in the right direction.
For instance, the data is out there to show us which approaches to building long-term brand equity deliver the most value. We know that TV, video on-demand, and print have the greatest long-term impact of all media channels. Whereas search, display, and radio ads tend to perform better in the short term. The problem is, if you’re only focused on those easy-to-measure short-term results, you’ll miss out on some significant opportunities.
Studies also show that it pays to run campaigns for longer, which may be another option overlooked if short-term returns aren’t immediately seen from an investment.
The real measure of success is the ability to balance insights into both the short and long-term impacts of your strategies. For decisions around where budgets should be allocated for a given category or segment – on primetime TV or Facebook, for instance – short-term insights are perfect for guiding your decision making, assuming they are regularly updated. For wider strategic changes to marketing campaigns and brand-focused advertising, long-term ROI has to be a key consideration.
In fact, Davide Fabrizio, Insight Analytics Director at Deloitte’s Analytics Unit, thinks the swing should move far more towards long-term insights:
“Think of 20-30% in the short term and 70-80% in the long term. Now more than ever it’s important to have measured the long-term impact of advertising in past historical data and to know the baseline of the business and the possible erosion that can occur by stopping advertising.”3
Client
A global food and beverage giant with 20+ brands that sell more than a billion products a day in over 200 countries and territories.
Challenge
Looking to fuel more robust, impactful, and data-driven marketing strategies, our client wanted insight into how its marketing mix impacted ROI. The ultimate goal was to make better use of annual budgets and optimise investments in the right marketing channels at the right time. The client was particularly interested in discovering the long-term impact of its marketing on brand equity.
Results
Using our AI+HI approach and a combination of internal and external data, two-staged econometric models and multivariate linear regression, we helped our client:
- Gain actionable insight into the overall ROI of media levers considering both short-term and long-term effects
- Add an additional 15-25% ROI through brand equity that a typical MMM methodology wouldn’t have identified
- Gain a clear view of optimum spend by channel, genre, and platform to inform future investment strategies
- Optimise $250+ million of media spend across all categories and markets
- Reallocate $3.5 million of marketing spend resulting in incremental 1% volume growth
A schematic of our approach – end-to-end market mixed solution using econometric models
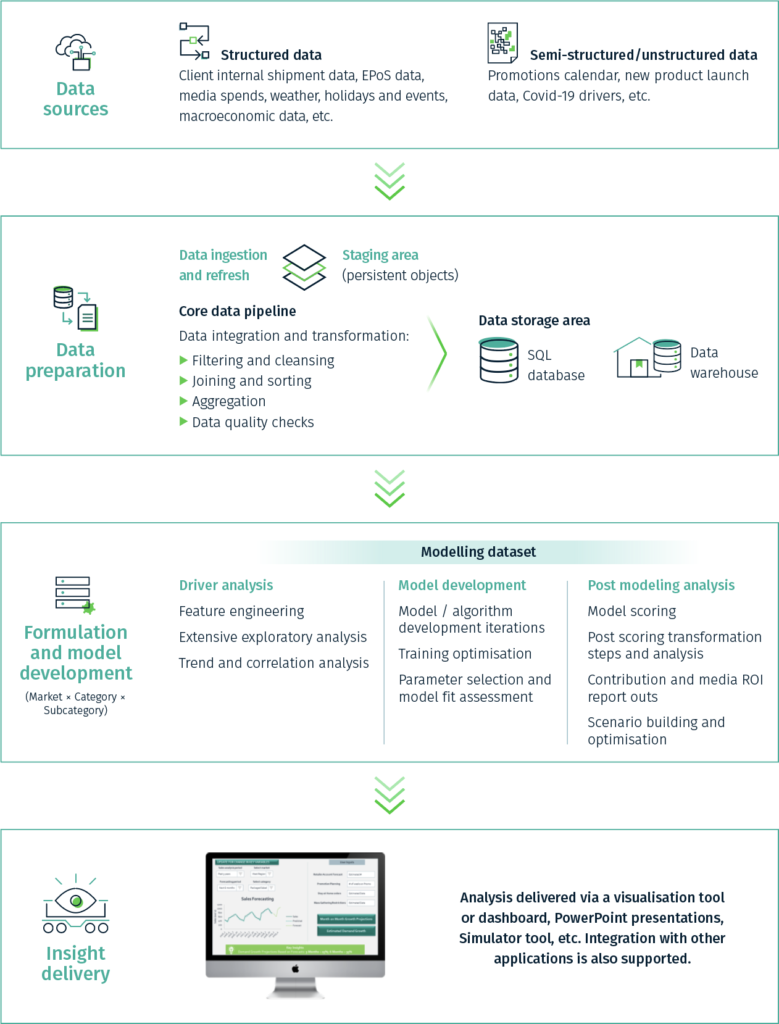
3. Adapting models for current market scenarios
If recent years have taught us anything, it’s that expecting the unexpected and having the ability to adapt quickly has become key to survival. Updating marketing mix models when the unexpected occurs and being able to understand how those events affect customer behaviour is a vital part of that.
The most prominent recent example of this, although not the only one by far, has been the COVID-19 pandemic. The various knock-on effects of the rapid spread of the coronavirus changed categories and consumer behaviours overnight. For instance, home-bound customers have led to a 60% increase in the amount of video content watched across the globe.4
Purchasing habits have changed, too. We personally saw the frozen food industry experience a huge spike in new customers, as more people were cooking from home. In this instance, a robust marketing mix analysis is needed for two reasons. Firstly, to prevent an update in sales from being misconstrued as the results of marketing investments. And secondly, to take advantage of the situation. To do the latter, you must have insight into the impacts of the pandemic. Media consumption will change; fewer people will be listening to the radio during their commute, for instance. And, conversely, TV and video on demand consumption will likely continue to grow as people spend more time at home.
Today’s technologies give companies the opportunity to build marketing mix models that can account for current economic conditions and sudden shifts, providing insights into long- and short-term impacts and how best to spend budgets. What makes this difficult to achieve is that MMM models typically lean on historical data to make predictions, which means models need to evolve to capture the impact of events in almost real-time. In addition, many models aren’t detailed enough to capture granular insights, like changes in regional impact or consumption patterns.
However, in examining these shortcomings it becomes easier to see how to build models that can predict the unique business impacts of changing consumer behaviour.
To be successful, a continuous cycle of analysing data, adapting strategies, and testing their results must be implemented to ensure market share is retained and new customers are on boarded.
Today’s technologies give companies the opportunity to build marketing mix models that can account for current economic conditions and sudden shifts
Use case
The Smart Cube helps a large frozen food manufacturer in Europe understand the impacts of COVID-19
Client
A large frozen food manufacturer in Europe, with a host of family favourite brands under its umbrella.
Challenge
When COVID-19 caused unprecedented spikes in frozen food purchasing, our client required new insights into commercial drivers such as price, consumer promotion, and media spend and how each affects volume.
As well as wanting to understand the short-term ROI of its campaigns and the halo impact of media spend across categories, the client had a special interest in understanding the specific impact COVID-19 had on demand, competitor activities, and media consumption.
Results
By collating a range of data from both internal and external sources, running detailed analysis to study data patterns and market dynamics, and carrying out feature selection techniques, we were able to help our client:
- Calculate the impact of the COVID-19 pandemic on sales volumes
- Conduct deep-dive analysis into the effectiveness of marketing campaigns by channel, and optimise £15 million in media spend
- Analyse two key competitor brands to gather intelligence on their strategies
- Deliver a 1% incremental volume increase through new synergies between media and promotions activities.
Conclusion
A Marketing Mix Modelling strategy is a must for organisations who want to optimise spend, grow market share, do more with shrinking budgets, or test hypothetical investments in a safe environment. And, importantly, it can be a powerful secret weapon when industries face unexpected disruption.
As our case studies have shown, the benefits of investing in such a solution can be huge – but for those big rewards, there needs to be a certain level of investment and support. Put simply, MMM can’t be an afterthought. It takes a team of skilled individuals, the right investments in data, and the desire to regularly examine, test, and alter strategies to be truly successful.
The good news is, the insights gained from these investments empower marketing teams, revenue managers, sales departments, analytics teams and more to do their jobs to the very best of their abilities.
It’s true that for many organisations, the resources might not exist to execute these strategies in-house, and we’ve already discussed the challenges this approach can present in terms of building and scaling operational models. By partnering with an experienced provider for your MMM, you can gain vital new perspectives, alongside the capabilities and resources you need on demand – so you only ever pay for what you use and can gain transformative insights when they are needed the most.
If you have any questions about this paper, or would like to learn what we can do to help you with your marketing mix strategy, get in touch.
Insights that drive continuous growthHowever you approach revenue growth management today, The Smart Cube can help you continuously uncover, understand, and assess the biggest opportunities in your markets. However you approach revenue growth management today, The Smart Cube can help you continuously uncover, understand, and assess the biggest opportunities in your markets. We leverage advanced AI and ML techniques to empower organisations to solve business challenges across a wide range of functions. Our solutions combine these technologies with the human insight, expertise, and contextual knowledge needed to drive the strongest results. That’s AI+HI, and it equals intelligence, accelerated. Using these techniques, we provide some of the world’s biggest companies with custom insights into their marketing mix strategies, helping them to optimise spend and turn hidden opportunities into successful investments. To learn more, visit our website |
-
Case Study 1
The Smart Cube helps a global food and drink leader gain ongoing insight into its marketing mix for snacks
-
Case Study 2
The Smart Cube helps a leading food and drink brand deliver 15-25% additional ROI through long-term insights into brand loyalty
-
Case Study 3
The Smart Cube helps a large frozen food manufacturer in Europe understand the impacts of COVID-19
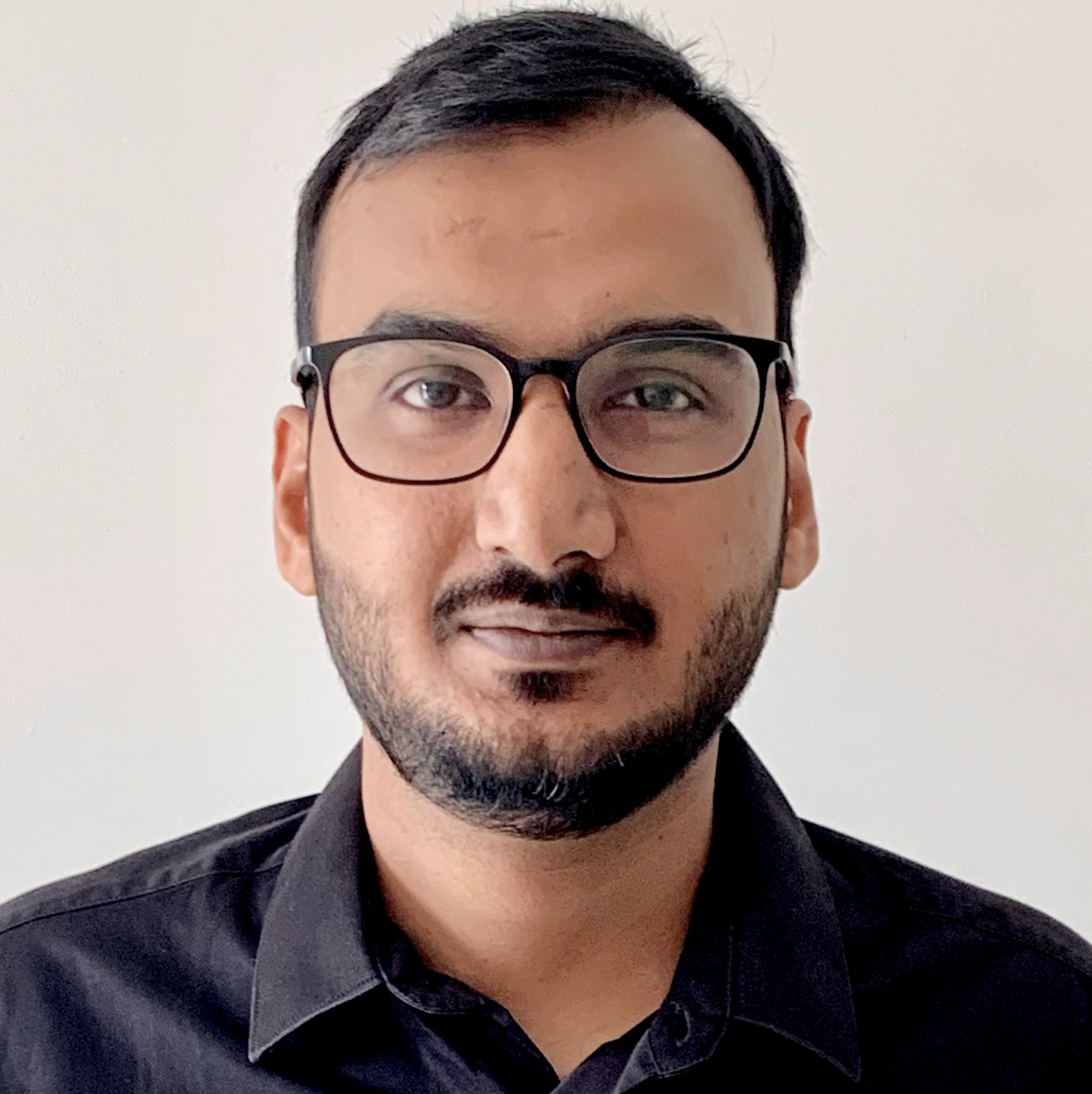
Ankur Jajodia an Advanced Analytics Professional, is a Senior Manager with over 12 years of experience in the customer and marketing analytics domain and has consulted numerous clients across CPG, Telecom, Hospitality and other industries with custom analytics solutions. A passionate advocate of driving business growth through data, Ankur constantly endeavours to create actionable solutions that help derive measurable business value. Over the past 12 years, he has helped 15+ clients in quantifying ROIs of their marketing investments and optimising budget allocation across traditional and digital media channels to boost future sales.
Ankur Jajodia
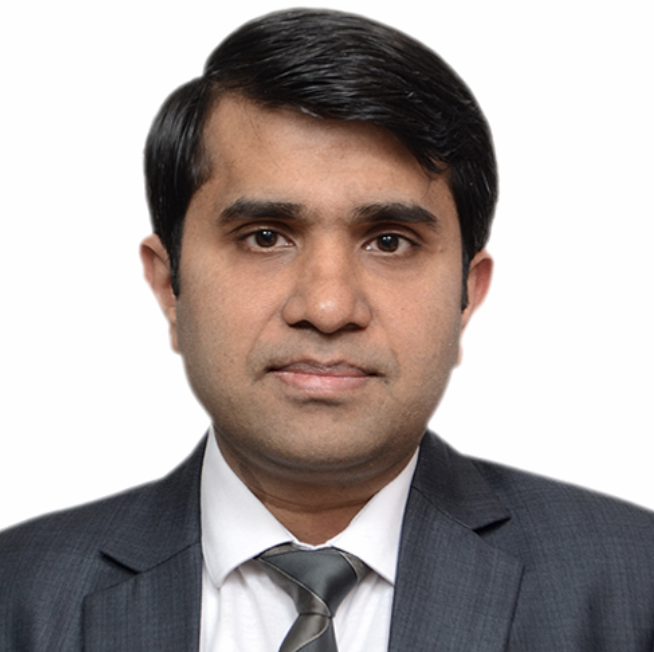
Vibhor Aggarwal is an analytics professional with 8 years of experience, primarily into Data Analytics focusing on exploratory/predictive study and its application in various facets of marketing spread across domains like CPG and Retail. He is adept in statistical modeling with experience in building Churn models, Attribution models, Market Mix models, Segmentation using various Statistical and Machine learning techniques like Logistic/Linear Regression, CHAID, Random Forest, K-means, to name a few.
Vibhor Aggarwal
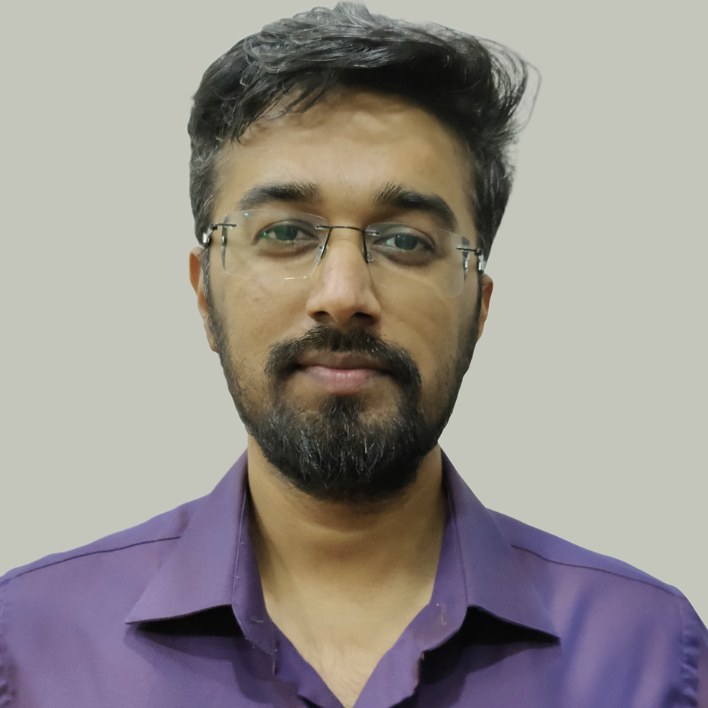
Aashish Aggarwal has 5 years of analytics experience in Data analytics, Statistical descriptive and predictive modelling in the marketing domain of CPG and Retail sectors. He excels in analysing data statistically and generating insightful observations using techniques like Market Mix Modelling, Clustering, Regression, Segmentation and Forecasting. He also likes to keep abreast of the latest developments and trends in analytics and artificial intelligence, to learn and develop new skills.
Aashish Aggarwal
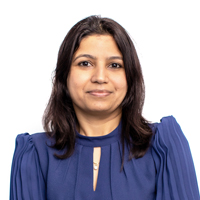
Nisha Purswani is an advanced data analytics and consulting professional with over 14 years’ experience in data-driven solutions for retail, CPG and pharmaceuticals industries. In her current role, Nisha is responsible for partnering with senior stakeholders from retail and CPG clients globally to develop data analytics strategy and design custom solutions to solve business problems using data science, analytics, and technology. When Nisha isn’t helping clients solve business problems, she can be found reading books, or in the kitchen trying out new recipes. She also enjoys travelling, meeting people of different cultures, and exploring new places.
Nisha Purswani